My North Star for the Future of AI
Length: • 13 mins
Annotated by howie.serious
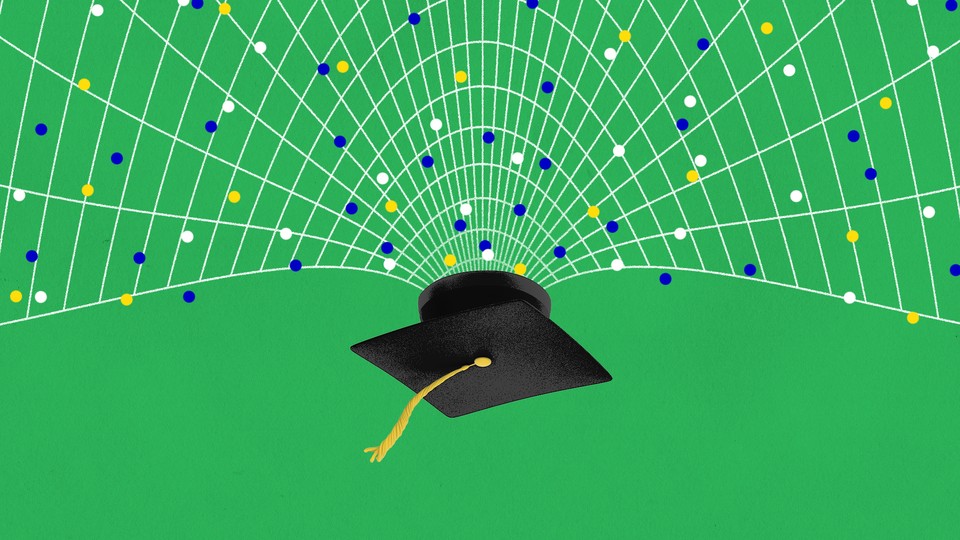
Whatever academics like me thought artificial intelligence was, or what it might become, one thing is now undeniable: It is no longer ours to control. As a computer science professor at Stanford, it had been a private obsession of mine—a layer of thoughts that superimposed itself quietly over my view of the world. By the mid-2010s, however, the cultural preoccupation with AI had become deafeningly public. Billboards along Highway 101 on the California coast heralded the hiring sprees of AI start-ups. Cover stories about AI fronted the magazines in my dentist’s waiting room. I’d hear fragments of conversation about AI on my car radio as I changed stations.
The little red couch in my office, where so many of the projects that had defined our lab’s reputation had been conceived, was becoming the place where I’d regularly plead with younger researchers to keep some room in their studies for the foundational texts upon which our science was built. I’d noticed, first to my annoyance and then to my concern, how consistently those texts were being neglected as the ever-accelerating advances of the moment drew everyone’s attention to more topical sources of information.
“Guys, I’m begging you—please don’t just download the latest preprints off arXiv every day,” I’d say. “Read Russell and Norvig’s book. Read Minsky and McCarthy and Winograd. Read Hartley and Zisserman. Read Palmer. Read them because of their age, not in spite of it. This is timeless stuff. It’s important.”
arXiv (pronounced “archive”) is an online repository of academic articles in fields such as physics and engineering that have yet to be published but are made available to the curious in an early, unedited form known as a “preprint.” The repository has been a fixture of university culture for decades, but in the 2010s, it became an essential resource for staying current in a field that was progressing so rapidly that everything seemed to change from one week to the next, and sometimes overnight. If waiting months for the peer-review process to run its course was asking too much, was it any surprise that textbooks written years, if not entire generations, before were falling by the wayside?
At the time, arXiv was just the start of the distractions competing for my students’ mindshare. More overtly, the hunt was already on as tech giants scrambled to develop in-house AI teams, promising starting salaries in the six-figure range, and sometimes higher, alongside generous equity packages. One machine-learning pioneer after another had departed Stanford, and even postdocs were on the menu by the middle of the decade. In one especially audacious episode, in early 2015, Uber poached some 40 roboticists from Carnegie Mellon University—all but decimating the department in the process—in the hopes of launching a self-driving car of its own. That was a hard-enough thing for my colleagues and me to witness. But for my students, young, eager, and still developing their own sense of identity, it seemed to fundamentally warp their sense of what an education was for. The trend reached its peak—for me, anyway—with an especially personal surprise. One of the computer scientists with whom I’d worked closest, Andrej Karpathy, told me he had decided to turn down an offer from Princeton and leave academia altogether.
“You’re really turning them down? Andrej, it’s one of the best schools in the world!”
“I know,” I remember him telling me. “But I can’t pass this up. There’s something really special about it.”
Andrej had completed his Ph.D. and was heading into what must have been the most fertile job market in the history of AI, even for an aspiring professor. Despite a faculty offer from Princeton straight out of the gate—a career fast track that any one of our peers would have killed for—he was choosing to join a private research lab that no one had ever heard of.
OpenAI was the brainchild of the Silicon Valley tycoons Sam Altman and Elon Musk, along with the LinkedIn co-founder Reid Hoffman and others, built with an astonishing initial investment of $1 billion. It was a testament to how seriously Silicon Valley took the sudden rise of AI, and how eager its luminaries were to establish a foothold within it. Andrej would be joining OpenAI’s core team of engineers.
From the September 2023 issue: Does Sam Altman know what he’s creating?
Shortly after OpenAI’s launch, I ran into a few of its founding members at a local get-together, one of whom raised a glass and delivered a toast that straddled the line between a welcome and a warning: “Everyone doing research in AI should seriously question their role in academia going forward.” The sentiment, delivered without even a hint of mirth, was icy in its clarity: The future of AI would be written by those with corporate resources. I was tempted to scoff, the way my years in academia had trained me to. But I didn’t. To be honest, I wasn’t sure I even disagreed.
Where all of this would lead was anyone’s guess. Our field has been through dramatic ups and downs; the term AI winter—which refers to the several-years-long plateaus in artificial-intelligence capabilities, and the drying up of funding for AI research that came with it—was born from a history of great expectations and false starts. But in the 2010s, things felt different. One term in particular was gaining acceptance in tech, finance, and beyond: the Fourth Industrial Revolution. Even accounting for the usual hyperbole behind such buzz phrases, it rang true enough, and decision makers were taking it to heart. Whether driven by genuine enthusiasm, pressure from the outside, or some combination of the two, Silicon Valley’s executive class began making faster, bolder, and, in some cases, more reckless moves than ever.
“So far the results have been encouraging. In our tests, neural architecture search has designed classifiers trained on ImageNet that outperform their human-made counterparts—all on its own.”
The year was 2018, and I was seated at the far end of a long conference table at Google Brain, one of the company’s most celebrated AI-research orgs, in the heart of its headquarters—the Googleplex—in Mountain View, California. The topic was an especially exciting development that had been inspiring buzz across the campus for months: “neural architecture search,” an attempt to automate the optimization of a neural network’s architecture.
A wide range of parameters defines how such models behave, governing trade-offs between speed and accuracy, memory and efficiency, and other concerns. Fine-tuning one or two of these parameters in isolation is easy enough, but finding a way to balance the push and pull between all of them is a task that often taxes human capabilities; even experts struggle to dial everything in just right. The convenience that automation would provide was an obviously worthy goal, and, beyond that, it could make AI more accessible for its growing community of nontechnical users, who could use it to build models of their own without expert guidance. Besides, there was just something poetic about machine learning models designing machine learning models—and quickly getting better at it than us.
But all that power came with a price. Training even a single model was still cost-prohibitive for all but the best-funded labs and companies—and neural architecture search entailed training thousands. It was an impressive innovation, but a profoundly expensive one in computational terms. This issue was among the main points of discussion in the meeting. “What kind of hardware is this running on?” one researcher asked. The answer: “At any given point in the process, we’re testing a hundred different configurations, each training eight models with slightly different characteristics. That’s a combined total of 800 models being trained at once, each of which is allocated its own GPU.”
Eight hundred graphics processing units. It was a dizzying increase. The pioneering neural network known as AlexNet had required just two GPUs to stop Silicon Valley in its tracks in 2012. The numbers grew only more imposing from there. Recalling from my own lab’s budget that the computing company Nvidia’s most capable GPUs cost something like $1,000 (which explained why we had barely more than a dozen of them ourselves), the bare-minimum expense to contribute to this kind of research now sat at nearly $1 million. Of course, that didn’t account for the time and personnel required to network so many high-performance processors together in the first place, and to keep everything running within an acceptable temperature range as all that silicon simmered around the clock. It doesn’t include the location either. In terms of both physical space and its astronomical power consumption, such a network wasn’t exactly fit for the average garage or bedroom. Even university labs like mine, at a prestigious and well-funded university with a direct pipeline to Silicon Valley, would struggle to build something of such magnitude. I sat back in my chair and looked around the room, wondering if anyone else found this as distressing as I did.
I had decided to take a job as chief scientist of AI at Google Cloud in 2017. Nothing I’d seen in all my years at universities prepared me for what was waiting for me behind the scenes at Google. The tech industry didn’t just live up to its reputation of wealth, power, and ambition; it massively exceeded it. Everything I saw was bigger, faster, sleeker, and more sophisticated than what I was used to.
The abundance of food alone was staggering. The breakrooms were stocked with more snacks, beverages, and professional-grade espresso hardware than anything I’d ever seen at Stanford or Princeton, and virtually every Google building had such a room on every floor. And all this before I even made my way into the cafeterias.
Next came the technology. After so many years spent fuming over the temperamental projectors and failure-prone videoconferencing products of the 2000s, meetings at Google were like something out of science fiction. Cutting-edge telepresence was built into every room, whether executive boardrooms designed to seat 50 or closet-size booths for one, and everything was activated with a single tap on a touchscreen.
Then there was the talent—the sheer, awe-inspiring depth of it. I couldn’t help but blush remembering the two grueling years it took to attract three collaborators to help build ambient intelligence for hospitals. Here, a 15-person team, ready to work, was waiting for me on my first day. And that was just the start—within only 18 months, we’d grow to 20 times that size. Ph.D.s with sterling credentials seemed to be everywhere, and reinforced the feeling that anything was possible. Whatever the future of AI might be, Google Cloud was my window into a world that was racing toward it as fast as it could.
I still spent Fridays at Stanford, which only underscored the different level Google was at, as word of my new position spread and requests for internships became a daily occurrence. This was understandable to a point, as my students (and the occasional professor) were simply doing their best to network. What worried me, though, was that every conversation I had on the matter, without a single exception, ended with the same plea: that the research they found most interesting wouldn’t be possible outside a privately run lab. Even at a place like Stanford, the budgets just weren’t big enough. Often, in fact, they weren’t even close. Corporate research wasn’t just the more lucrative option; it was, more and more, the only option.
Finally, there were the data—the commodity on which Google’s entire brand was based. I was surrounded by them—and not just by an indescribable abundance but data in categories I hadn’t even imagined before: from agriculture businesses seeking to better understand plants and soil, from media-industry customers eager to organize their content libraries, from manufacturers working to reduce product defects, and so much more. Back and forth I went, as the months stretched on, balancing a life between the two institutions best positioned to contribute to the future of AI. Both were brimming with talent, creativity, and vision. Both had deep roots in the history of science and technology. But only one seemed to have the resources to adapt as the barrier to entry rose like a mountain towering over the horizon, its peak well above the clouds.
Read: The future of AI is GOMA
My mind kept returning to those 800 GPUs gnawing their way through a computational burden that a professor and her students couldn’t even imagine overcoming. So many transistors. So much heat. So much money. A word like puzzle didn’t capture the dread I was beginning to feel.
AI was becoming a privilege. An exceptionally exclusive one.
Since the days of ImageNet, the database I’d created that helped advance computer vision and AI in the 2010s, it had been clear that scale was important—but the notion that bigger models were better had taken on nearly religious significance in recent years. The media was saturated with stock photos of server facilities the size of city blocks and endless talk about “big data,” reinforcing the idea of scale as a kind of magical catalyst, the ghost in the machine that separated the old era of AI from a breathless, fantastical future. And although the analysis could get a bit reductive, it wasn’t wrong. No one could deny that neural networks were, indeed, thriving in this era of abundance: staggering quantities of data, massively layered architectures, and acres of interconnected silicon really had made a historic difference.
What did it mean for the science? What did it say about our efforts as thinkers if the secret to our work could be reduced to something so nakedly quantitative? To what felt, in the end, like brute force? If ideas that appeared to fail given too few layers, or too few training examples, or too few GPUs suddenly sprang to life when the numbers were simply increased sufficiently, what lessons were we to draw about the inner workings of our algorithms? More and more, we found ourselves observing AI empirically, as if it were emerging on its own. As if AI were something to be identified first and understood later rather than engineered from first principles.
The nature of our relationship with AI was transforming, and that was an intriguing prospect as a scientist. But from my new perch at Google Cloud, with its bird’s-eye view of a world evermore reliant on technology at every level, sitting back and marveling at the wonder of it all was a luxury we couldn’t afford. Everything that this new generation of AI was able to do—whether good or bad, expected or otherwise—was complicated by the lack of transparency intrinsic to its design. Mystery was woven into the very structure of the neural network—some colossal manifold of tiny, delicately weighted decision-making units, meaningless when taken in isolation, staggeringly powerful when organized at the largest scales, and thus virtually immune to human understanding. Although we could talk about them in a kind of theoretical, detached sense—what they could do, the data they would need to get there, the general range of their performance characteristics once trained—what exactly they did on the inside, from one invocation to the next, was utterly opaque.
An especially troubling consequence of this fact was an emerging threat known as “adversarial attacks,” in which input is prepared for the sole purpose of confusing a machine learning algorithm to counterintuitive and even destructive ends. For instance, a photo that appears to depict something unambiguous—say, a giraffe against a blue sky—could be modified with subtle fluctuations in the colors of individual pixels that, although imperceptible to humans, would trigger a cascade of failures within the neural network. When engineered just right, the result could degrade a correct classification like “giraffe” into something wildly incorrect, like “bookshelf” or “pocket watch,” while the original image would appear to be unchanged. But though the spectacle of advanced technology stumbling over wildlife photos might be something to giggle at, an adversarial attack designed to fool a self-driving car into misclassifying a stop sign—let alone a child in a crosswalk—hardly seemed funny.
Granted, more engineering might have helped. A new, encouraging avenue of research known as “explainable AI,” or simply “explainability,” sought to reduce neural networks’ almost magical deliberations into a form humans could scrutinize and understand. But it was in its infancy, and there was no assurance it would ever reach the heights its proponents hoped for. In the meantime, the very models it was intended to illuminate were proliferating around the world.
Even fully explainable AI would be only a first step; shoehorning safety and transparency into the equation after the fact, no matter how sophisticated, wouldn’t be enough. The next generation of AI had to be developed with a fundamentally different attitude from the start. Enthusiasm was a good first step, but true progress in addressing such complex, unglamorous challenges demanded a kind of reverence that Silicon Valley just didn’t seem to have.
Read: We don’t actually know if AI is taking over everything
Academics had long been aware of AI’s negative potential when it came to issues like these—the lack of transparency, the susceptibility to bias and adversarial influence—but given the limited scale of our research, the risks had always been theoretical. Even ambient intelligence, the most consequential work my lab had ever done, would offer ample opportunities to confront these pitfalls, as our excitement was always tempered by clinical regulations. But now that companies with market capitalizations approaching a trillion dollars were in the driver’s seat, the pace had accelerated radically. Ready or not, these were problems that needed to be addressed at the speed of business.
As scary as each of these issues was in isolation, they pointed toward a future that would be characterized by less oversight, more inequality, and, in the wrong hands, possibly even a kind of looming, digital authoritarianism. It was an awkward thought to process while walking the halls of one of the world’s largest companies, especially when I considered my colleagues’ sincerity and good intentions. These were institutional issues, not personal ones, and the lack of obvious, mustache-twirling villains only made the challenge more confounding.
As I began to recognize this new landscape—unaccountable algorithms, entire communities denied fair treatment—I concluded that simple labels no longer fit. Even phrases such as out of control felt euphemistic. AI wasn’t a phenomenon, or a disruption, or a puzzle, or a privilege. We were in the presence of a force of nature.
What makes the companies of Silicon Valley so powerful? It’s not simply their billions of dollars, or their billions of users, or even the incomprehensible computational might and stores of data that dwarf the resources of academic labs. They’re powerful because of the many uniquely talented minds working together under their roof. But they can only harness those minds—they don’t shape them. I’d seen the consequences of that over and over: brilliant technologists who could build just about anything but who stared blankly when the question of the ethics of their work was broached.
The time has come to reevaluate the way AI is taught at every level. The practitioners of the coming years will need much more than technological expertise; they’ll have to understand philosophy, and ethics, and even law. Research will have to evolve too.
The vision I have for the future of AI is still tied together by something important: the university. AI began there, long before anyone was making money with it. Universities are where the spark of some utterly unexpected research breakthrough is still most likely to be felt. Perceptrons, neural networks, ImageNet, and so much since have come out of universities. Everything I want to build already has a foothold there. We just need to put them to use.
This, collectively, is the next North Star: reimagining AI from the ground up as a human-centered practice. I don’t see it as a change in the journey’s direction so much as a broadening of its scope. AI must become as committed to humanity as it’s always been to science. It should remain collaborative and deferential in the best academic tradition, but unafraid to confront the real world. Starlight, after all, is manifold. Its white glow, once unraveled, reveals every color that can be seen.
This article has been adapted from Fei-Fei Li’s new book, The Worlds I See: Curiosity, Exploration, and Discovery at the Dawn of AI.
Some content could not be imported from the original document. View content ↗