Antibodies are the product of evolution on two timescales: over...
Length: • 3 mins
Annotated by Ilias
Antibodies are the product of evolution on two timescales: over millions of years in vertebrate populations, and within weeks of infection or ๐ in populations of B cells. We ask how those timescales interact in our paper out in @PLOSPathogens ๐งต 1/n t.ly/U84Un
Antibodies start out as receptors on the surface of B cells, produced by the stochastic recombination of germline immunoglobulin genes that diversified over millions of years. This diversity allows the pool of newly produced ("naive") B cells to bind virtually any antigen. 2/n
Activated B cells divide and give rise to multiple lineages competing for antigen. Their initial fitness depends on the set of germline genes encoding each receptor, and we know that some genes/alleles are more likely than others to encode high-affinity receptors. 3/n
However, B cell lineages can subsequently evolve within the body under selection for mutations that improve antigen binding, a process known as affinity maturation. As they do so, they might overcome germline-encoded fitness differences. 4/n
Which lineages win out, what sites they target and how well they do so shapes the antibody response. In simulations and experiments, we ask how much these ecological and evolutionary outcomes depend on the initial choice of germline genes vs. subsequent B cell evolution. 5/n
This question informs the predictability of the antibody response: If B cells with specific germline genes consistently outcompete the others, we expect similar gene frequencies in the response of different individuals. 6/n
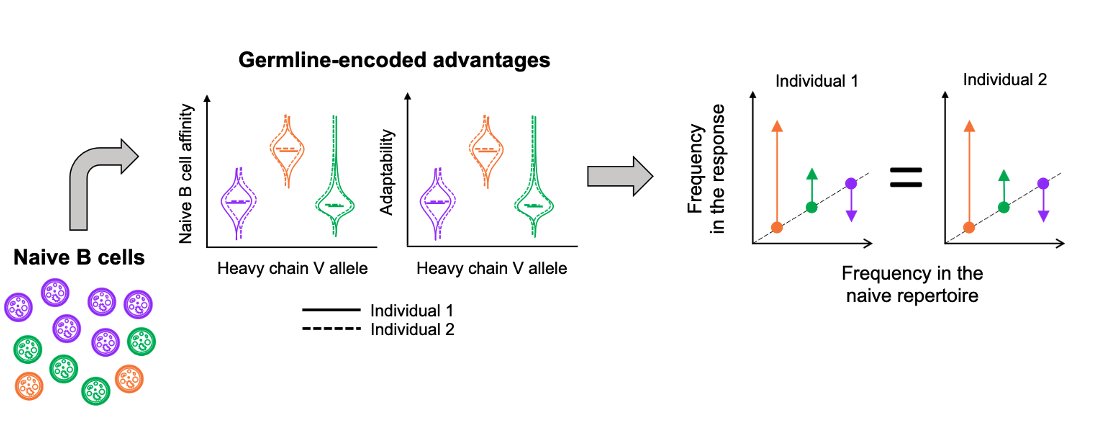
But if fitness depends more strongly on subsequent evolution than on the initial germline genes, chance events will likely cause lineages using different genes to win out in different individuals, leading to genetically different B cell responses between them. 7/n
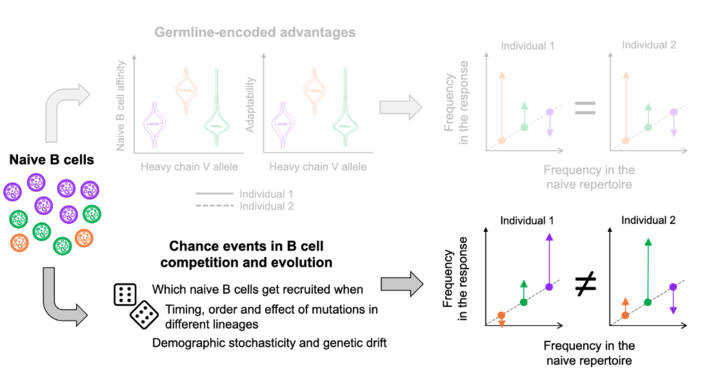
In simulations, we found that germline-encoded fitness differences can lead to either transient or persistent similarity between individuals, depending on how large the initial differences are relative to the effect of mutations introduced during affinity maturation. 8/n
When the initial advantage is large relative to the effects of mutation, B cells using those high-affinity germline genes consistently dominate the response across individuals, leading to similar gene frequencies between them. 9/n
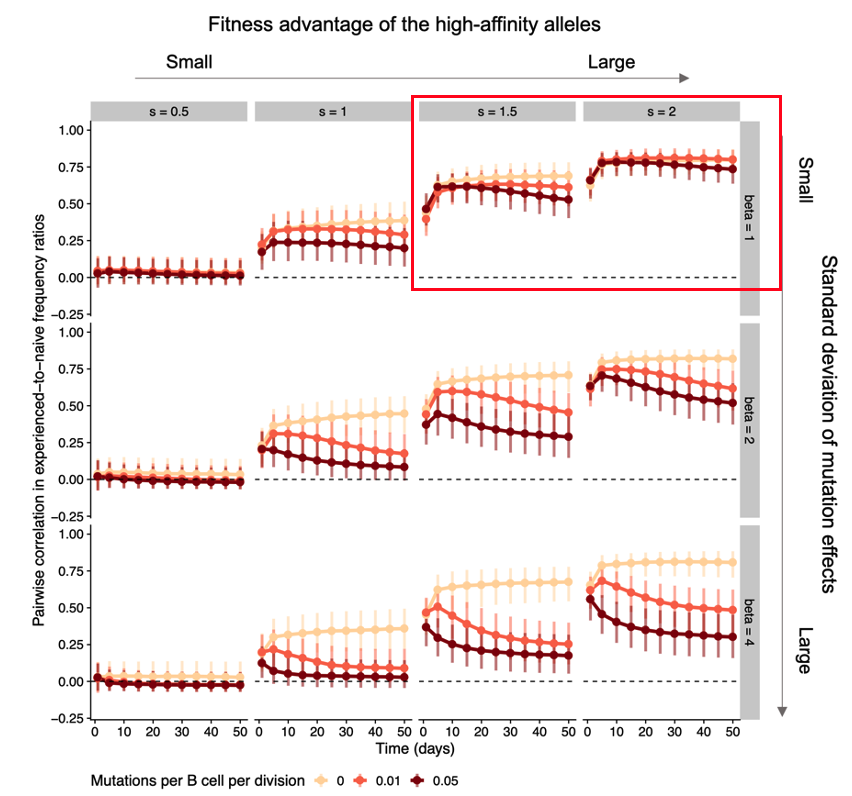
Weaker initial advantages lead to similar responses early on but are later overcome by B cell evolution playing out differently in each individual due to chance events (historical contingencies, as Stephen J. Gould put it), leading to decreasing similarity 10/n
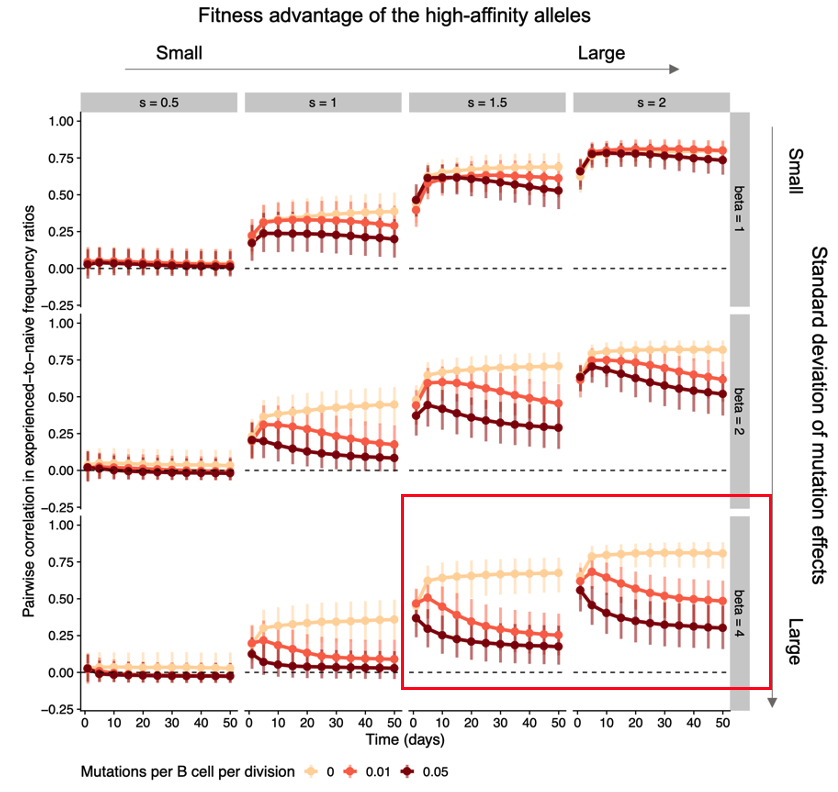
When do we expect germline-encoded specificities to be weak or strong? They might start out relatively weak if they arise by chance as evolutionary spandrels (Gould again), byproducts of the long-term diversification of immunoglobulin genes. 11/n frontiersin.org/article/10.338...
We find patterns consistent with this possibility in mice infected with flu, a pathogen that doesn't naturally infect them and could not nave selected for germline-encoded specificities: some genes consistently dominate early on but individual responses diverge over time. 12/n
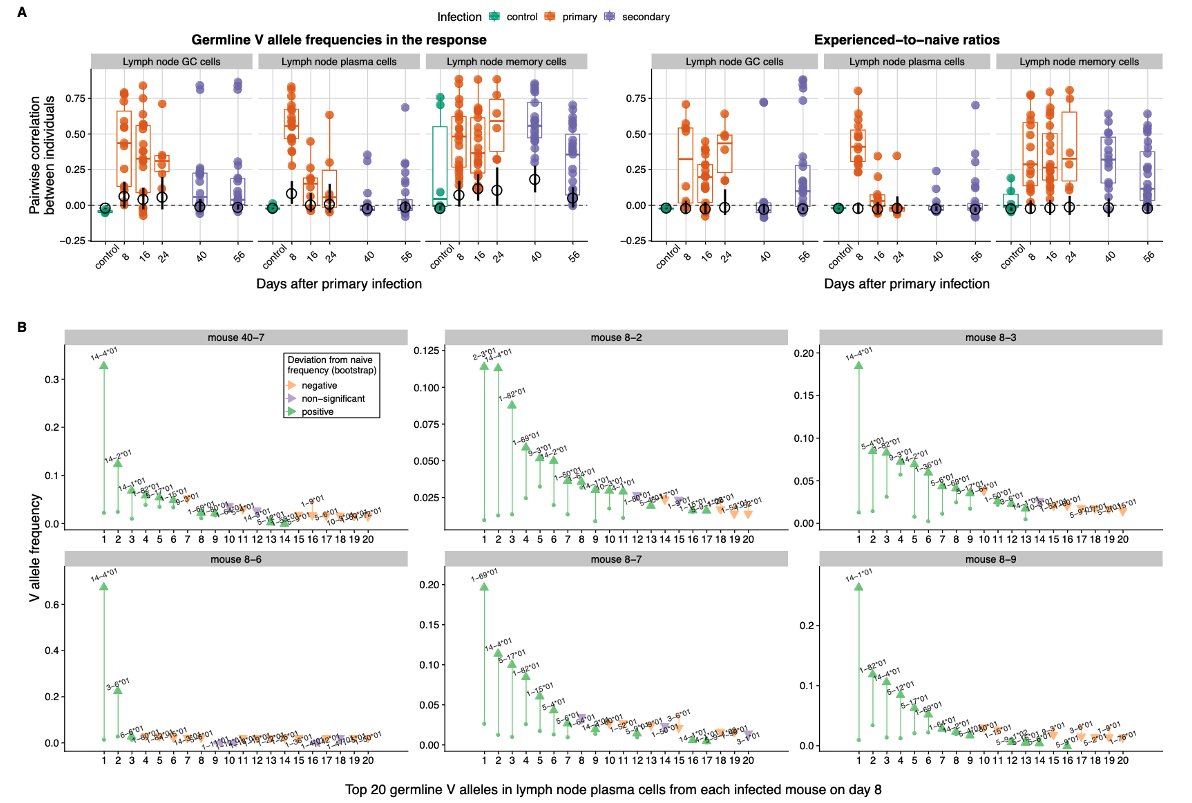
If improving the early response via hardcoded specificities increases host fitness, selection might reinforce those specificities, which our results suggest would lead to persistently similar responses across individuals. 13/n
Our findings in mice contrast with many reports of genetically similar responses across individuals ("public clones"). The difference might be partly due to our focus on the whole flu virus instead of specific epitopes, but this remains to be tested. 14/n
We hope this work will help us understand the degree of "convergence" or "publicness" in antibody responses (which is key to some vaccination strategies) and the long-term evolution of immunoglobulin genes and adaptive immunity. 15/n
@sarahcobey and I thank our fantastic collaborators, who are former/current members of the Boyd (@ScottBoydLab) and Wilson (@PatWilsonLab1) labs: @DoctorAKP, @Stamperion, Micah Tepora, Khoa Nguyen and Tho D. Pham. We also thank 4 (!) thoughtful reviewers.