0 - 1M ARR in 7 months
Length: • 14 mins
Annotated by Ayush Chaturvedi


⏱ We launched on 29th July 2022. It took us 178 days to get to $1m ARR, and 217 days to get to the ramen-profitable state.
🌎 We have grown to ~30 people now distributed across 3 continents, 4 countries, and 6 cities.
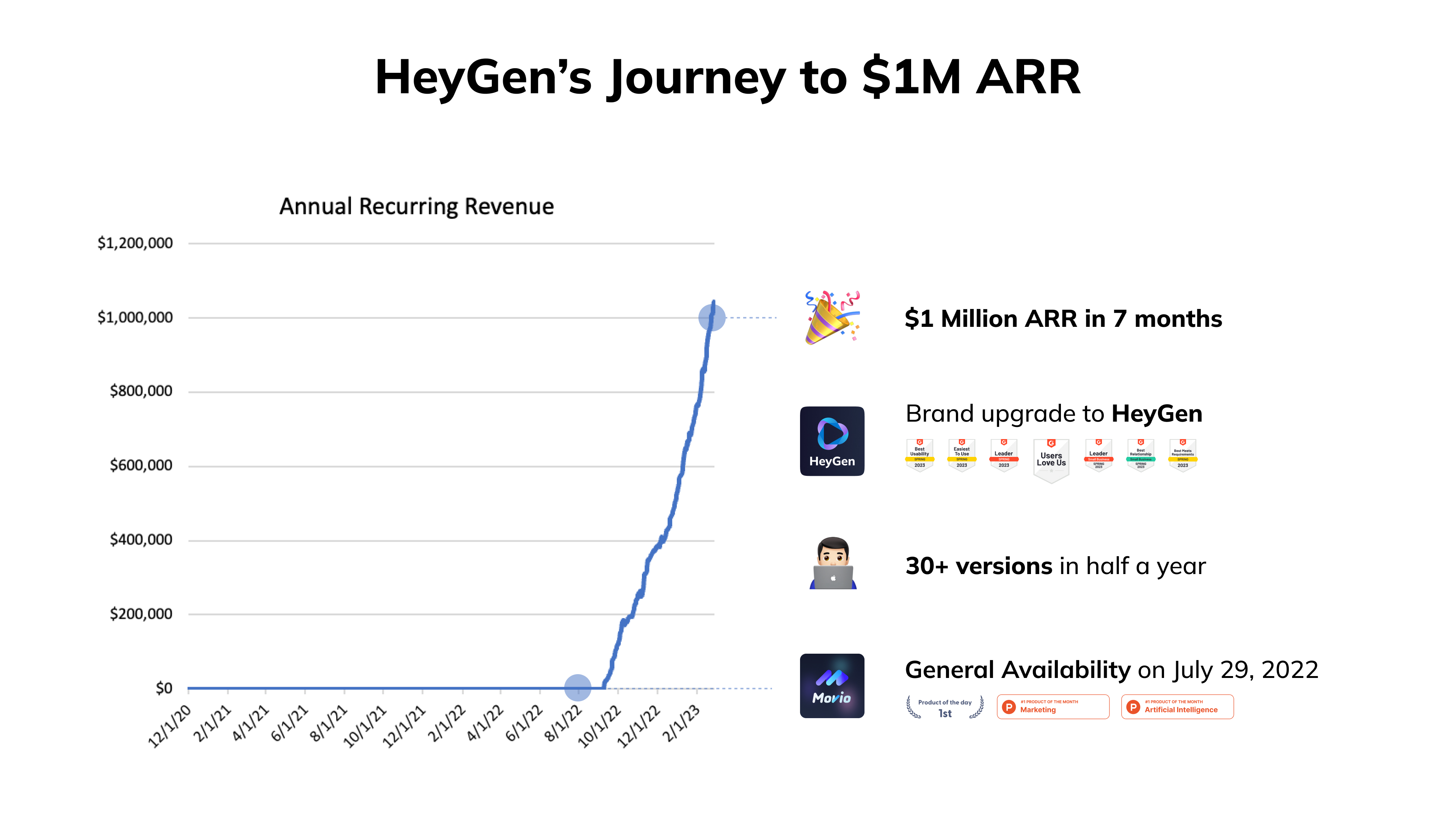
Here's a look at our numbers. Of course, we are not the fastest, but we hope this serves as an example of the future and growth possibilities.
It was a fantastic journey looking back. Wayne and I come from a consumer product background (Snapchat + Smule). We didn't have much experience with SaaS 12 months ago. But we figured that it was a necessary path to pursue our vision. So we just did it. We've learned a lot by just reading + talking to different people with prior experience. I am so grateful that many people have helped us, and I would like to start this post to give back to the community, especially to founders in the AI space, sharing the story of how we hit $1M ARR.
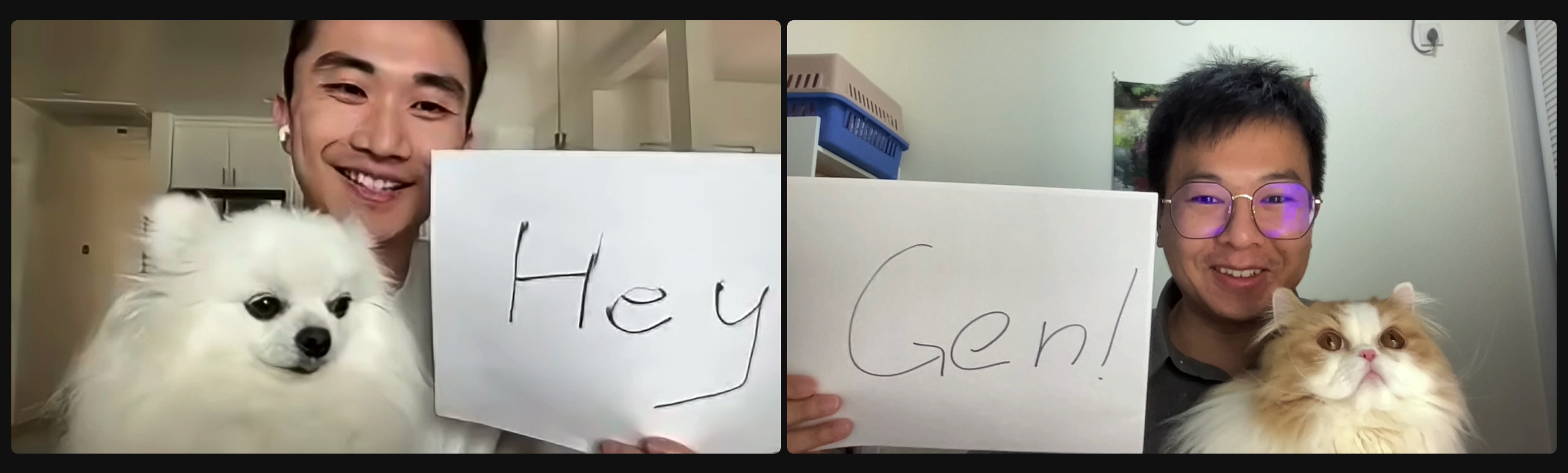
I will share how we found our first paying customer, how we validated the PMF, Zoomed into our product development journey, how we built products, how we worked with customers, and lastly, how we learned.
PMF -> AI-Market-Fit
I have a lot of experience in building AI models, hacking, and product development. However, none of these skills matter when you don't have a product-market fit (PMF). This is especially crucial in today's AI boom. I'd like to share another concept we implemented internally - AI-market-fit.
In the face of emerging technologies, distinguishing between demo values and user values can be challenging. Many technology demos appear incredibly cool, and I agree (I was once an avid enthusiast myself). But in the long run, demo values diminish quickly, and only user values remain.
When we founded the company in December 2020, the concept of generative AI was still a long way from becoming mainstream. Nonetheless, we were convinced from the beginning that AI could generate high-quality content. Our ultimate goal was to revolutionize visual storytelling by developing a visual engine that would transition from a traditional camera-based approach to AI generation.

To achieve our vision, we broke it down into three steps. The first step is to build a video engine for business. Next, we set an initial milestone of creating a SaaS product, allowing us to implement our technology, explore its boundaries, establish relationships with users, and simultaneously generate revenue to continue investing in the long run.

After a period of exploration and considering both technical feasibility and use cases, we identified the spokesperson scenario as our target market. However, a critical question arose: how could we validate whether this was a genuine AI-market fit?
The answer was Fiverr.
There were 1,811 available services on Fiverr when we searched for "spokesperson." If we could develop our idea into a great product experience, there would be a market for it, and people would be willing to pay for our solution.

Exactly 10 months ago, we set up a Fiverr account and launched a gig, offering on-demand video footage in multiple languages. At that time, we already had an early version of our technology. While it wasn't perfect, it was adequate for testing market demand. We didn't disclose that our avatars were AI-generated in the initial version. Instead, we provided the same services as other actors on Fiverr, but at just 10% of the cost, with a turnaround time of 10 minutes instead of days. I would manually run code to deliver videos to customers.
Our competitive pricing and rapid delivery helped us stand out in the Fiverr marketplace, leading to our first paying customer for a mere $5. Afterward, we updated our gig description to reveal that AI created our avatars, and our services remained popular. We quickly attracted a few more customers (30+), allowing us to understand use cases and pricing expectations better. We discovered that people were willing to pay $3 per minute for spokesperson videos.
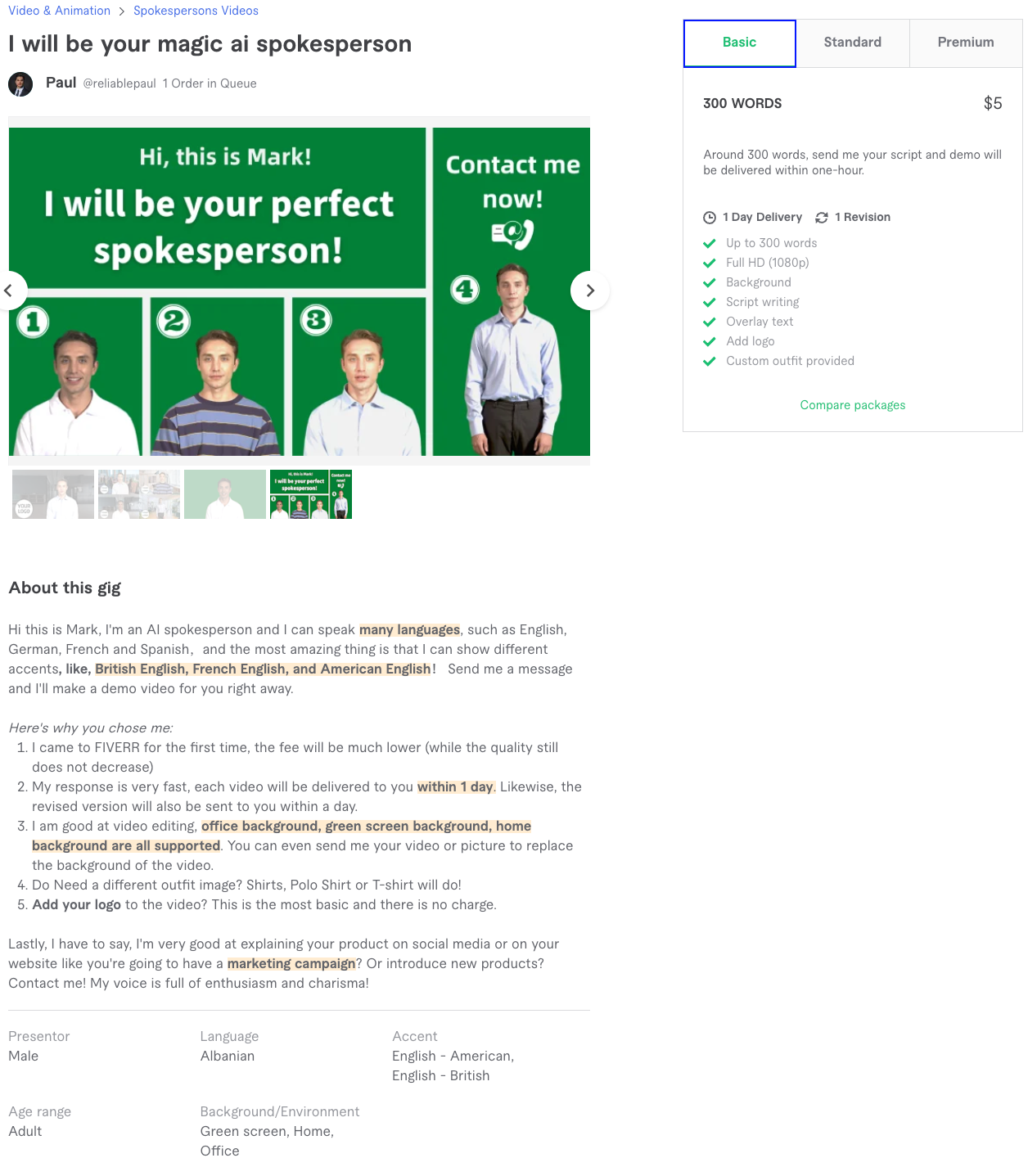
This allowed us to validate the AI-market-fit we were looking for at a minimal cost without building a full-fledged product. As a result, we acquired our first paying customer. More importantly, this initial group of users later became customers of our product, providing valuable feedback and a solid foundation for our first version. For almost every efficiency tool, we can verify whether similar market patterns exist on platforms like Fiverr or Upwork. These platforms represent the world of demand and supply. We can find numerous ideas such as translation, SEO articles, image creation, video production, voiceovers, and more.
Lesson learned
- Talk to the right person as early as possible, even before building the product. Find a way to develop a minimum viable product (MVP) quickly and have users pay for it; if they don't pay, it doesn't count.
- After meeting your real users, listen to their perspectives like you know nothing before. In reality, it turns out to be much more difficult than you think to resist thinking you know what they want based on your experience and so-called visions. It takes practice to really forget what you think your users need to internalize what they really want. As a technical founder, I've had many ideas about how new technology "can" help, but in most cases, they don't work. One great example is our CG avatar.
- We were part of the metaverse hype, where everyone believed CG humans and the metaverse would soon be the future. I also looked into this technology, thinking it was cool and could be a valuable addition to our existing product. However, it didn't result in a good product-market fit, became difficult to maintain, and needed more user adoption. Ultimately, we had to pause the service and let go of the team. Although the tech was excellent, only a few users needed it. People wanted more original and engaging personal videos. We could have avoided this issue by prioritizing the user's perspective.
- Avoid wishful thinking, especially for tech founders. I've made many mistakes as new technologies emerge daily, but the inherent value of technology can sometimes be quite distant from achieving product-market fit.
- Finding the right problem is far more important than solving the current "most important" problem. When you get it right, it's an entirely different experience than working on a fake product-market fit. You can smell it.
Product Journey
Launch on Jul 29th
We began developing the product in Q2 2022 and launched on July 29th, 2022. I still remember the excitement on the day of our Product Hunt launch. We successfully secured the #1 Product of the Month in AI and the #1 Product of the Month in Marketing. That milestone began HeyGen's (previously Movio) incredible journey.

Inventing TalkingPhoto
When explaining to customers how to create their avatar, a frequently asked question is, "Can I use a photo?" The answer was no. However, this question strongly indicated that some users 1) wanted a low-cost way to create spokesperson videos and 2) desired a quick method to test the feature before committing to filming the complete footage.
As a result, we innovated by developing the ability to make a photo talk, which we call a "TalkingPhoto." The feature received excellent feedback during beta testing, and it has been one of the key factors in our successful product-led growth (PLG) strategy. TalkingPhotos are fun, engaging, and creative, which results in extensive social sharing. The recent viral "Balenciaga/Harry Potter" example perfectly demonstrates its appeal.
Ramping up traffic - freemium + watermark, causing 3 outages in a month
We approached product-led growth (PLG) by applying various consumer product growth strategies, where Wayne and I are more experienced, to the B2B context. To begin with, we chose freemium over a free trial. The next question was how to encourage more sharing. While we implemented a typical method of allowing users to invite friends for referral credits, which worked well, we wanted to do something more innovative as a video company.
The answer we found was simple but highly effective: freemium + a strong watermark. I agree that our watermark is more prominent than usual, but that's what we needed for the initial bootstrap. In social apps, people calculate the viral coefficient for user acquisition by counting how many new users are invited by existing users. Although we don't have the same friending network effect as social apps, our content generates a strong 'viewing-sharing' network effect. If we have to pinpoint what we've done to acquire users, this network effect is the only strategy we employed, and we achieved a "viral coefficient" (number of new customers / number of new watermark videos) of more than 3.0.
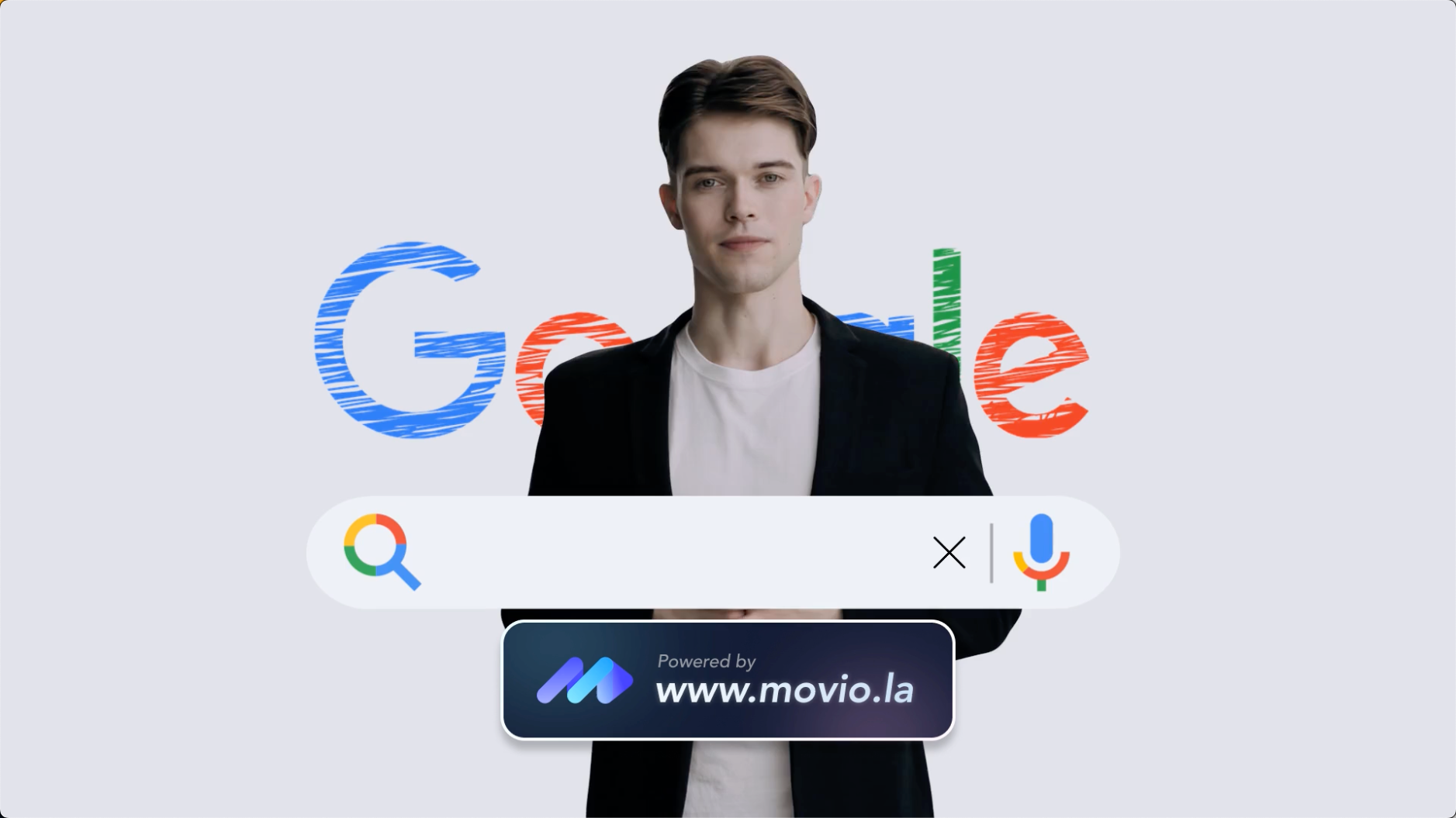
Many people say that for every SaaS product, 80% of the traffic comes from one channel. This is our channel, and we've optimized the entire workflow through fine-tuning and experimentation.
We launched the freemium + watermark combo on September 12th, along with the Stripe paywall. As a result, several user-generated videos received millions of views on social media, causing our system to crash a few times. (which was a good problem to have.)
Amplify the viral engine - Gen AI Map Featuring
We started to gain significant traction in the industry and began to get featured on numerous Generative AI maps. The first mention came from Sequoia Capital on October 24th. Since then, we've had many mentions and features on social media. With the built-in viral momentum, we continue to amplify our user acquisition engine.
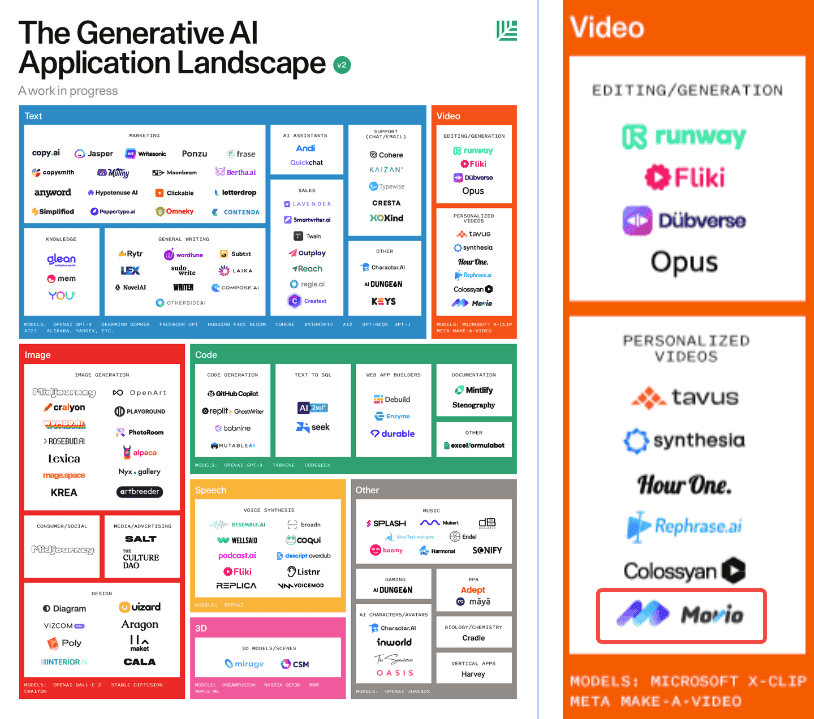
Improving customer experience
Initially, our product was an entirely new concept for many customers. So a lot of them didn't actually get activated. So we spent three months, from November to January, focusing on just the onboarding experience.
By continuously talking to customers and watching them try the product step by step, we concluded that the first aha moment in their journey was not them trying their first template, playing around with our AI avatars, or using video tools, but instead watching their first AI-generated video. So the customer experience quickly became the primary focus for the whole team. We then explored every possible way to help customers get to the aha moment faster, including:
- Building landing pages that allow users to create a video without even registering for an account
- Embedding onboarding videos upon successful registration (these videos are made using our product)
- Creating hundreds of templates for different use cases to expedite the user's content creation process (we built 200 templates in just one week with only two people)
In the end, we managed to double the conversion rate since November. Also, we received almost 200 reviews on G2 with a solid 4.8/5.0 rating.
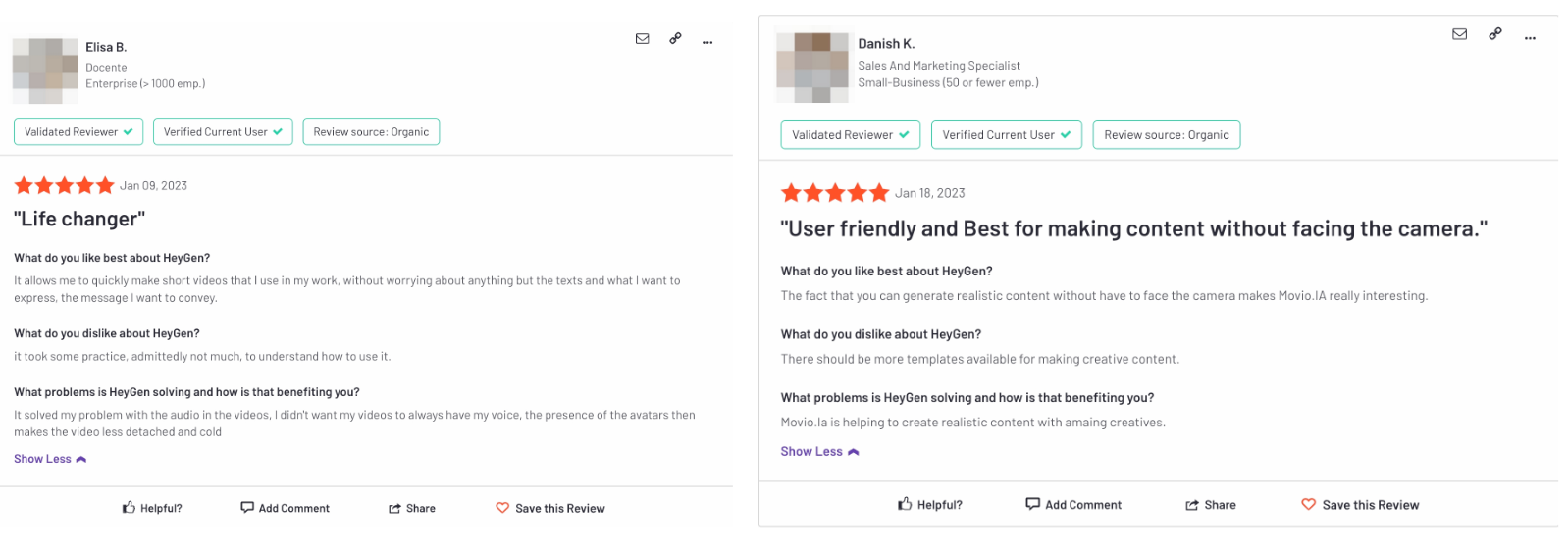
How We Build the Product?
We have a lot of experience in building a product from a small company to a big company. Our perspective on product development may differ from that of larger organizations. Still, we've found that it works well for startups.
Weekly release
We have maintained a weekly release schedule since we launched the product. Doing this is a difficult task, where at most fast-paced internet companies, a bi-weekly release is standard. But we find it to be beneficial on the path to reaching $1M ARR, which not only speeds up product iteration but also forces us to prioritize the most important things. There isn't time for secondary issues.
How we did it - we would usually finalize the design for the upcoming week's development on Sundays. Then, releases would happen on Thursdays to provide a buffer to fix any issues on Fridays. Meanwhile, on Fridays, we would also do an initial data analysis for the new release and a more in-depth analysis of the prior week's release (which had been live for a week by then). We kept repeating this process every week. A single Airtable sheet is all we used to manage everything.
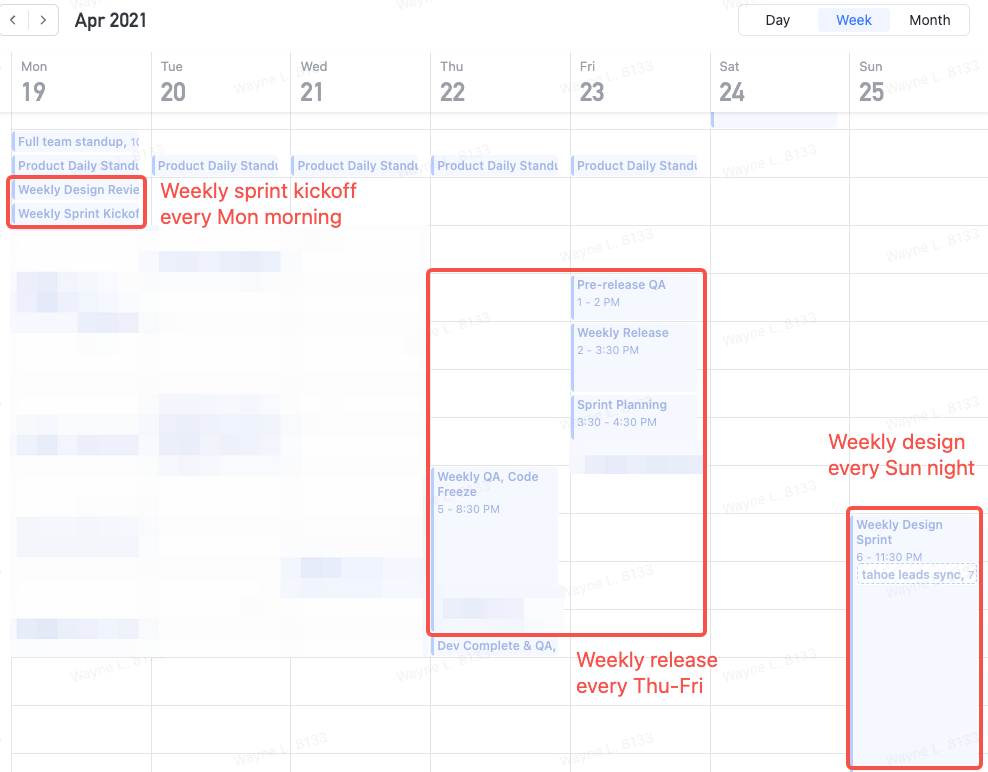
However, the cost of doing this was that things would break. And we took the hit.
Fix it only when it breaks
It might be counter-intuitive, but we only optimize or fix something until it breaks, which is acceptable early because we can move fast enough to fill the gap. Meanwhile, this approach helps us avoid premature optimizations. In the early stage of AI-Market-Fit, most optimizations are unnecessary because of the inherent uncertainties in demand and product features. As a result, we might be optimizing the wrong problem. Then, when a problem must be fixed, we start seeing the occurrence of AI-Market-Fit. For us, there were a few waves of traffic in Sep/Oct that caused our server to crash for a couple of hours – that's the AI-Market-Fit moment. When you find AI-Market-Fit, your server will begin to crash, which is by design. We can maximize the number of iterations with this method.
Another unconventional perspective is that the only way to verify your PMF is if someone breaks it. Design your system so that if real PMF occurs, it will fail. The goal is to get things out quickly and test as many ideas as possible. Having things break means something is right.
For example, we use a single MySQL for our database. We could pick a more sophisticated DB for better scalability, but why? Just use MySQL and let it break. If fewer than 1,000 people are using your product, a MySQL instance will be more than enough, and it probably means the PMF is not there yet. We also barely wrote any unit tests in the early stage.
We applied the same mindset to other areas as well. For example, we didn't use anything fancy for project management like JIRA, Asana, or Trello. Instead, we relied on a single Airtable sheet to manage everything. It wasn't perfect, but it got the job done.
Adapting to distributed teamwork
Initially, I assumed remote teams might lag behind onsite teams in productivity, especially on a local scale. However, I discovered that the main bottleneck often lies in the interactions and dependencies among different team components, such as when one team is blocked by another.
To address these challenges, we implemented several key practices:
- Embrace asynchronous communication: Using tools like Loom encourages team members to focus on the main points and allows viewers to watch at an accelerated speed, enhancing communication efficiency.
- Accommodate different time zones: Scheduling at least two regular 30-min meetings at the intersection of two time zones helps ensure that team members across the globe stay connected and informed.
- Hire a flexible team: A team willing to adapt their schedules for occasional early-morning or late-night meetings can maintain momentum and speed in a fast-moving startup.
Implementing these strategies has improved productivity and allowed us to provide a phenomenal experience for our users, e.g., 24/7 support and customer calls, all-day service availability without worrying about stability, and fast growth across different countries.
Build a data dashboard as early as possible
We use metabse as our visualization engine for our DB layer. We have 200+ dashboards in total. We also use Python notebooks to build more sophisticated saas metrics, e.g., https://sacks.substack.com/p/the-saas-metrics-that-matter I highly recommend building all these data dashboards and picking up whatever makes the most sense for you to optimize. Build it before releasing the product; build it even when the data is wrong. We can always come back to fix it.
AB testing is a trap
In the early stages of a startup, A/B testing may not be the most effective approach. It can be seen as over-engineering since insufficient data would produce statistically significant results. Instead, ship fast. If there are issues, address them based on feedback and make the necessary adjustments.
Tools we used
We leveraged numerous third-party tools to help us bootstrap our project quickly and efficiently by not reinventing the wheel. Here's a subset of the tools we used:
- Datadog (we didn't use Grafana) + PagerDuty
- Azure Synapse (for managed data workflows; during the first six months, we essentially overwrote the entire analytical DB from MySQL every day, without managing incremental update pipelines)
- Shotstack (to bootstrap our initial video rendering pipeline)
- Zapier (to automate every possible workflow across the team)
- Typeform + Calendly (not just for scheduling but also to build a mini CRM in conjunction with Zapier)
- Webflow + Jasper
- Intercom
- Amplitude
- Unlimited ChatGPT Plus and GPT-4 API access for everyone in the company
- And more
- Remember that many of the strategies discussed here might only apply to the early stage of a startup, particularly before reaching 1 million ARR. After that point, procedures may need to be adjusted accordingly.
- Additionally, it's crucial to build only a few features at the outset. If we had to start over, we would focus more on one key feature and would have launched the product three months earlier. For example, the product could have been launched without the editor view. We could have focused only on green screen high-quality video generation. This would have helped us reach the market faster with more time to gather user feedback.
Customers
In our experience, the key to building a great product lies in fostering solid product-user momentum. With this, we don't have to decide which features to build; instead, we should gather customer feedback and let that guide our priorities.
We discussed how we found our initial AI-Market-Fit with Fiverr. In this section, I will focus on how we've grown alongside our customers.
Maximize how many customers we can talk to
While we often hear advice that we should listen to customers, I'd like to share some concrete facts about how we do that. First of all, every user can schedule a call with us without even registering an account.
Over the past 217 days, we've conducted 1,400 customer Zoom meetings, averaging seven daily meetings. Wayne and I have participated in 800 of those meetings, taking notes during each one and sharing them with the entire team.
During these calls, it is essential to let the customer talk. (Check out this post for effective customer interviews: https://www.youtube.com/watch?v=z1iF1c8w5Lg)
We've divided our Intercom support into three time zones, maximizing our ability to chat with customers and gather valuable insights. (Our goal isn't to provide 24/7 support but to learn from our customers as much as possible.)

Build transparency with the team
We maintain two chat groups: "HeyGen Loves" and "HeyGen Hates", to capture ALL customer feedback. Usually, "HeyGen Hates" is busier because the team is eager to identify areas for improvement. During all-hands meetings, we prioritize sharing customer feedback before discussing any metrics. Customer input always comes first!
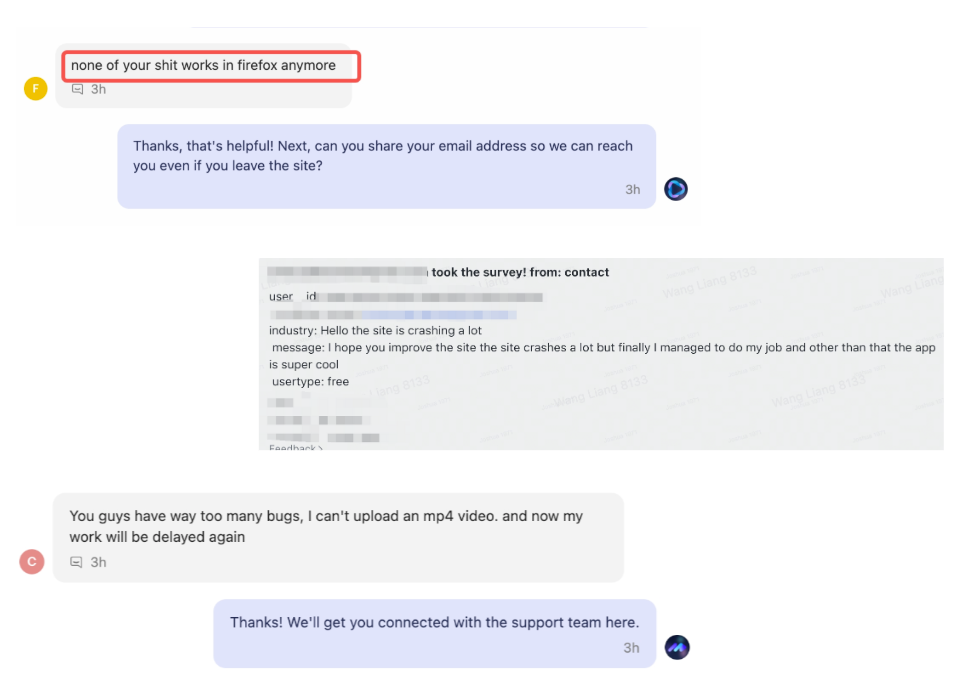
Avoid customization
Resist the temptation to accept customization requests, even with a large check. Instead, evaluate whether the proposed solution would benefit just one customer or if it would be advantageous for all customers.
How we do things that don't scale
We often hear about the importance of this approach. This is what we did for customer success. To track our customers, we use a simple Airtable sheet, an approach we learned from Andreas Klinger (https://klinger.io/posts/the-simplest-and-most-important-dashboard-for-early-stage-startups). Internally, we only consider video generation activity as retention because that links directly to the user values we are creating.
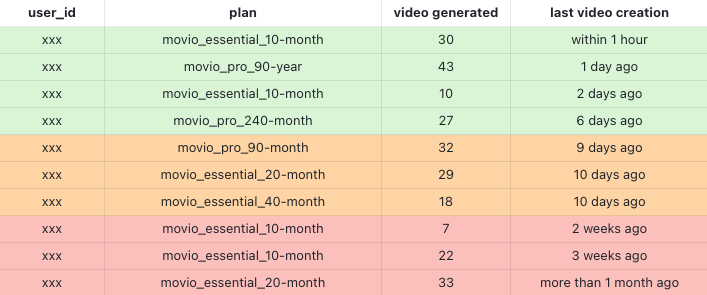
This is the first thing I check every morning, and our goal is to maximize the ratio of green. I believe there's no magic behind it; many excellent product managers could achieve this by prioritizing customer success. However, this focus allows us to concentrate on the most critical aspect - creating user value.
We continued this approach until we reached over 1,000 customers. That's when we needed to start thinking about scaling.
Learning to build a SaaS product has been an enjoyable experience, and it's quite different from building a consumer product. As a founder, you often need to strike a balance between two extremes. In my experience, it's crucial to be confident yet humble in order to learn quickly. Be confident in your ability to learn anything, as long as you invest time and effort into it. At the same time, remain extremely humble, acknowledging that you might be wrong, someone else may have a better idea, and should steal from the winners first.
Here are a few insights into how I learn:
- I have listened to every single SaaStr podcast episode since 2020, while driving to work.
- Substack has been a fantastic learning resource, such as Lenny's Newsletter, Kyle Poyar's Growth Unhinged.
- Studying other SaaS products, competitors, or even products in different areas teaches me a lot.
- Have an adviser. It is essential to ensure I learn the right way. Learn not only from their advice, but most importantly, how they arrived at them. Compare my approach with theirs, and ask why they would not choose mine. It is like the RLHF.
Beyond $1M ARR
As of the date of this blog post (April 26th), I am thrilled to share that HeyGen has achieved yet another significant milestone - we are on track to be profitable this month! 2 months ago, we celebrated our $1M ARR achievement, and I am immensely grateful for our dedicated team and every customer who has supported us along the way. Our success would have been impossible without your support.
On the product front, we are currently developing HeyGen 2.0, which will feature additional team collaboration and enterprise capabilities. Additionally, we've accumulated millions of video data which allows us to build vertical LLM applications for video, which will unblock the end-to-end video generation experience.
There is still a lot of room for growth and improvement in our go-to-market motion. As such, we are actively expanding our GTM team and hiring top talent in this area. There will be more things we can learn, and I am really looking forward to sharing our story with you all on our $10M ARR milestone.
Building a startup can be challenging, sometimes painful, but also rewarding. I hope our journey can inspire and assist you in your startup endeavors.
Thank you!